Main Research Goals
In ViRAPID we will overcome the limitations of current approaches for simulating photo-induced reactions on long time scales by applying rare event sampling together with Tully's fewest switches surface-hopping approximation for non-adiabatic dynamics in combination with machine learning potentials based on neural networks.
As we have recently shown, machine learning potentials allow evaluating the potential energy surfaces entering the surface hopping approach much faster and yet accurately [1]. Thus, ViRAPID will allow investigating the mechanism and calculate rate constants of complex non-adiabatic reactions involving rare barrier crossing events for the first time.
In order to accelerate the discovery of photoreaction mechanisms, ViRAPID will implement a unique code based on rare event sampling with non-adiabatic surface-hopping and neural networks for electronic excited states.
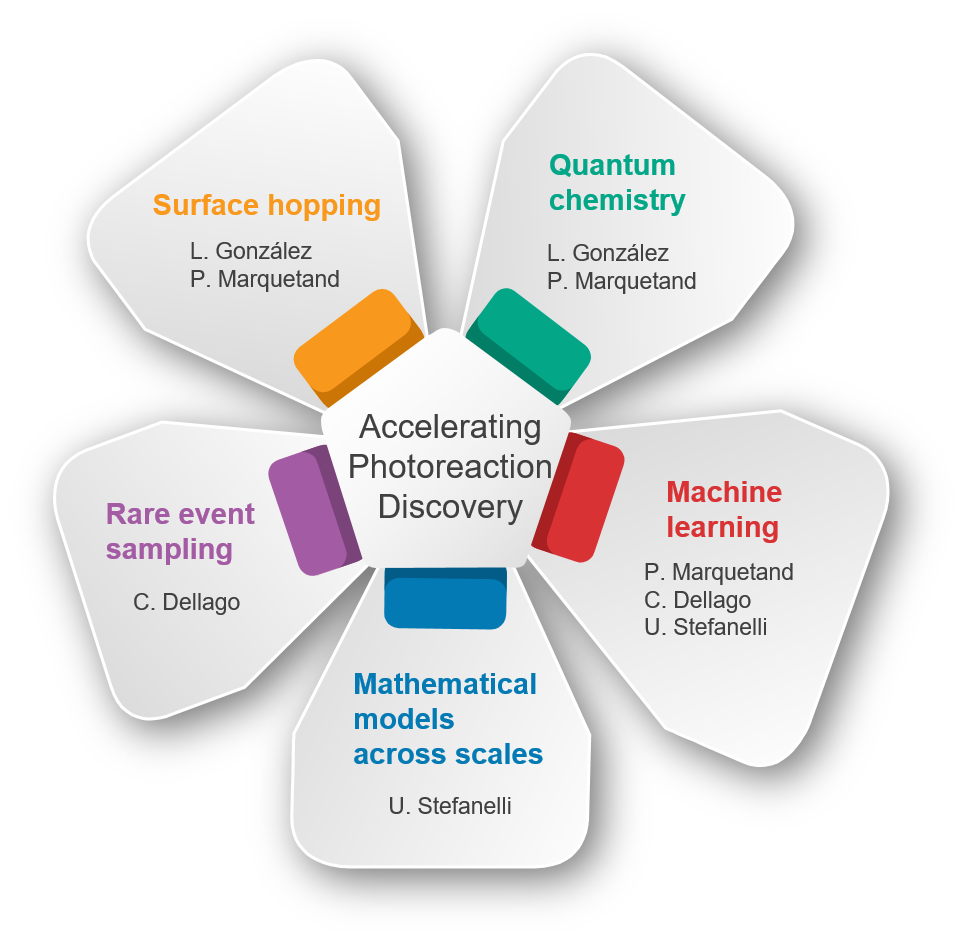
Such combined methodology will be able to bridge the length and timescales associated with the simulation of photoreactions and requires the expertise of complementary teams across three faculties for its successful implementation: L. González / Chemistry (expert on electronic excited-state dynamics with a focus on surface hopping and quantum chemistry), P. Marquetand / Chemistry (expert on machine learning for ground and excited-state dynamics), C. Dellago / Physics (expert on rare event sampling) and U. Stefanelli / Mathematics (expert on mathematical modelling in materials science across scales).
[1] J. Westermayr, M. Gastegger, M. Menger, S. Mai, L. González, P. Marquetand
Machine learning enables long time scale molecular photodynamics simulations
Chem. Sci., 10, 8100-8107 (2019).